The Rise of White Label AI in SaaS Ecosystems
The software-as-a-service (SaaS) sector is undergoing a fundamental transformation, thanks to artificial intelligence integration. White label AI SaaS solutions have emerged as game-changers, allowing businesses to offer sophisticated AI capabilities under their own brand without developing the technology from scratch. This shift is particularly significant as companies seek competitive advantages in crowded markets. According to Gartner research, AI-enhanced SaaS applications are projected to grow by 34% annually through 2025, outpacing traditional SaaS models. The customizable nature of white label solutions allows startups and established enterprises alike to deploy conversational AI, voice agents, and automated customer service tools without massive R&D investments. The conversational AI for medical offices is just one example of how these technologies are transforming specific industries with specialized AI implementations.
Understanding White Label AI: The Framework for Innovation
White label AI represents a business model where developers create AI systems that other companies can rebrand as their own. Unlike traditional software licensing, these solutions provide complete branding flexibility while maintaining sophisticated backend functionality. The core components typically include natural language processing engines, machine learning algorithms, voice synthesis capabilities, and customizable interfaces. This framework offers significant advantages in terms of speed-to-market and cost efficiency. For instance, implementing AI voice agents through white label solutions costs approximately 60-80% less than building proprietary systems. These platforms commonly provide API access, allowing seamless integration with existing business systems like CRMs, help desks, and e-commerce platforms. Companies like SynthFlow AI have pioneered this approach, enabling businesses to quickly deploy customized AI solutions across diverse applications from sales to customer support.
Why Businesses Are Gravitating Toward White Label AI Solutions
The business case for white label AI adoption is compelling across multiple dimensions. First, the development timeline for custom AI systems typically spans 12-18 months, while white label implementations can be completed in weeks. Second, the financial equation heavily favors white labeling—custom AI development often requires investments of $500,000+ before generating revenue, whereas white label solutions operate on predictable subscription models with minimal upfront costs. Third, businesses can maintain brand consistency across all customer touchpoints while leveraging cutting-edge AI technology. Fourth, these solutions offer remarkably quick paths to revenue generation, particularly in areas like AI appointments scheduling and automated customer engagement. Fifth, the flexibility to scale operations based on demand without corresponding infrastructure investments presents a significant operational advantage. Companies utilizing AI calling for business have reported 40-60% reductions in customer acquisition costs while increasing conversation rates by 25-30%, according to recent industry benchmarks.
The Technical Architecture Behind White Label AI SaaS
The technological foundation of white label AI SaaS platforms consists of several sophisticated layers that work together seamlessly. At the core, these systems utilize large language models (LLMs) like GPT-4, Claude, or DeepSeek to understand and generate natural language. This capability is enhanced through specialized voice synthesis engines from providers such as ElevenLabs or Play.ht that convert text responses into natural-sounding speech. The communication layer typically leverages telephony infrastructure through services like Twilio or affordable SIP carriers, allowing AI agents to make and receive calls. These components are integrated through robust APIs and middleware that handle everything from call routing to speech-to-text processing. What makes white label solutions particularly valuable is their packaging of these complex technologies behind user-friendly configuration interfaces, allowing non-technical teams to customize AI behaviors, responses, and workflows without coding knowledge. The AI call center white label solutions exemplify how these technical components can be packaged for specific industry applications.
Key Market Players in the White Label AI SaaS Space
The white label AI SaaS marketplace features several prominent players, each with distinct specializations and competitive advantages. Retell AI has established itself as a pioneer in voice agent technology, offering sophisticated conversation capabilities for customer service applications. Bland AI has gained traction with its focus on natural-sounding voice synthesis and seamless telephony integration. Air AI differentiates through its specialized sales automation features and CRM integrations. Vapi AI has carved out a niche with developer-friendly APIs and extensive customization options. Callin.io itself has emerged as a comprehensive platform combining voice AI, appointment setting, and customer engagement tools with white label capabilities. Beyond these specialized providers, major cloud platforms like AWS, Google Cloud, and Microsoft Azure offer white-labeled AI components that enterprises can incorporate into broader SaaS offerings. This competitive landscape continues to evolve rapidly, with new entrants regularly introducing innovations in voice quality, conversation handling, and integration capabilities.
Case Study: Transforming Call Centers with White Label AI
The call center industry provides a compelling illustration of white label AI’s transformative impact. Traditional call centers face persistent challenges: high agent turnover (typically 30-45% annually), escalating labor costs, and inconsistent customer experiences. By implementing AI call center technologies through white label solutions, businesses have achieved remarkable operational improvements. A mid-sized insurance company deployed white-labeled voice AI agents to handle tier-one customer inquiries, resulting in 67% faster resolution times, 24/7 availability without staffing concerns, and a 42% reduction in operational costs within six months. The technology handled over 80% of routine inquiries autonomously while seamlessly escalating complex cases to human agents. Similarly, a telecommunications provider implemented call center voice AI through white label solutions, which processed 12,000+ customer interactions daily with 93% satisfaction ratings—comparable to their best human agents. These implementations demonstrate how white label AI solutions enable companies to rapidly deploy sophisticated AI capabilities without extensive technical expertise or development resources.
The Economics of White Label AI: Revenue Models and ROI
The financial dynamics of white label AI present compelling business opportunities for both providers and implementers. For SaaS companies offering white label AI solutions, the typical revenue structure includes tiered subscription models based on usage volume (calls processed, minutes used) or feature access. These providers commonly achieve 75-85% gross margins due to the scalable nature of their platforms. For businesses implementing white labeled AI, the return on investment equation is equally attractive. The upfront costs typically involve platform subscription fees ($500-5,000 monthly depending on scale), integration expenses, and customization efforts. The payback period for these investments generally ranges from 4-8 months across industries. For example, companies using AI sales representatives report 35-50% reductions in customer acquisition costs while simultaneously increasing conversion rates. An e-commerce retailer implementing white labeled AI voice assistant for FAQ handling reduced support ticket volume by 62% within three months, translating to annual savings of $340,000. These economics explain the rapid adoption across industries from healthcare to financial services.
Industry Applications: Where White Label AI Makes the Biggest Impact
White label AI solutions have demonstrated particularly strong adoption across several key sectors. In healthcare, AI calling bots for health clinics handle appointment scheduling, medication reminders, and basic patient inquiries with 24/7 availability. Real estate agencies utilize AI calling agents to qualify leads, schedule property viewings, and maintain regular client follow-ups without expanding staff. E-commerce companies implement AI voice assistants to reduce cart abandonment rates by proactively engaging customers experiencing checkout difficulties. Professional services firms deploy AI receptionists to manage appointment scheduling and initial client screening. Financial services organizations use conversational AI for loan pre-qualification and account servicing. The common thread across these applications is the ability to deliver personalized, responsive service at scale without corresponding increases in personnel costs. Industries with high customer interaction volumes, routine inquiry patterns, and appointment-based business models have realized the most significant operational benefits from white label AI implementations.
Customization Capabilities: Beyond Off-the-Shelf Solutions
A defining characteristic of advanced white label AI platforms is their extensive customization potential. These systems offer multi-dimensional personalization far beyond simple branding elements. Voice personalization allows businesses to select specific voice profiles, adjust speech patterns, and sometimes create synthetic voices that align with brand identity. Conversation flow customization enables detailed scripting of AI interactions, including branching logic, response variations, and personality traits that reflect brand values. Integration flexibility permits connections with existing business systems like CRMs, payment processors, and scheduling tools. Industry-specific knowledge bases can be incorporated to ensure AI agents possess relevant terminology and process understanding. Some platforms even support prompt engineering that allows non-technical users to refine AI behavior without coding. The most sophisticated white label offerings provide dashboard analytics that track performance metrics and conversation outcomes. This customization depth allows businesses to create distinctive AI experiences that feel purposefully designed rather than generic implementations, significantly enhancing customer engagement and satisfaction levels.
Integration Challenges and Solutions for White Label AI
While white label AI offers tremendous potential, successful implementation requires addressing several common integration challenges. Data synchronization between AI systems and existing business applications presents a frequent hurdle, particularly with legacy systems lacking modern APIs. Leading providers address this through pre-built connectors for popular platforms and flexible webhook implementations for custom integrations. Voice quality optimization across varying network connections requires specialized handling, with adaptive bitrate technologies and buffering systems ensuring consistent experiences. Call routing logic that seamlessly blends AI and human agents demands sophisticated workflow design, usually implemented through visual editors that define escalation paths and handoff protocols. Security and compliance considerations, especially for SIP trunking and voice data, necessitate end-to-end encryption and regional data processing options to meet regulations like GDPR or HIPAA. API rate limiting and concurrent call handling must be carefully configured to prevent system overloads during peak periods. Providers like Callin.io have developed onboarding protocols specifically designed to address these integration points systematically, typically achieving full deployment within 2-3 weeks even for complex enterprise environments.
The User Experience Dimension: Making AI Feel Natural
Creating AI interactions that feel natural rather than robotic represents a critical success factor for white label implementations. Current-generation platforms have made significant advances in this area through several techniques. Dynamic conversation handling allows AI agents to maintain context throughout interactions, remember previous statements, and respond naturally to topic changes. Natural language understanding capabilities correctly interpret customer intent even with colloquial expressions, regional dialects, or industry jargon. Emotional intelligence features detect sentiment through voice tone analysis and adjust responses accordingly. Brief, natural pauses and hesitations in speech patterns create more human-like conversations compared to instantaneous responses. The best implementations also include personalized references to previous interactions, seamless handling of conversation interruptions, and appropriate humor or personality traits. Companies utilizing AI call assistants have found that customers often cannot distinguish between advanced AI agents and human representatives, with user satisfaction ratings comparable to or exceeding those of traditional call centers. This natural interaction quality significantly impacts customer perception of brands deploying white label AI solutions.
The Reseller Opportunity: Building AI-Powered SaaS Businesses
White label AI has created lucrative opportunities for entrepreneurial resellers who can package, customize, and market these solutions to specific industries or business segments. The AI reseller model enables companies to enter the rapidly growing AI market without technical development capabilities. Successful resellers typically focus on well-defined vertical markets—such as healthcare practices, legal firms, or real estate agencies—where they understand specific pain points and workflows. The economic model is particularly attractive, with typical reseller margins ranging from 30-50% while requiring minimal infrastructure investment. Many resellers enhance value through industry-specific customizations, integration services, and ongoing support packages. For example, agencies starting AI calling services can leverage white label platforms to offer fully managed communication solutions to local businesses. This approach allows relatively small organizations to deliver sophisticated AI capabilities that would otherwise require enterprise-scale development resources. The most successful resellers combine technical configuration expertise with deep industry knowledge, positioning themselves as specialized consultants rather than mere technology providers.
Data Privacy and Ethical Considerations in White Label AI
As AI-powered communications become more prevalent, data privacy and ethical implementation have emerged as critical considerations. White label AI platforms must address several key concerns to maintain trust and compliance. Conversation data handling policies need explicit protocols for storage duration, encryption standards, and usage limitations. GDPR and CCPA compliance requirements impact everything from consent mechanisms to data subject access rights. Transparency regarding AI usage is increasingly important, with many jurisdictions requiring disclosure when customers interact with automated systems rather than humans. Bias mitigation in AI training data helps prevent discriminatory outcomes across different demographic groups. While white label solutions simplify technical implementation, they don’t eliminate the reseller’s responsibility for ethical deployment. Leading platforms address these concerns through features like anonymization options for sensitive data, configurable retention policies, clear disclosure templates, and regional data processing controls. Companies utilizing these systems for sales calls or customer service must carefully balance automation benefits with appropriate human oversight and intervention capabilities.
Future Directions: Where White Label AI SaaS Is Heading
The trajectory of white label AI SaaS points toward several significant developments in the coming years. Multimodal interaction capabilities will expand beyond voice to integrate video, messaging, and web interfaces into seamless omnichannel experiences. Advanced personalization will leverage customer history and preferences to dynamically adjust AI conversation styles and recommendations in real-time. Industry-specific AI models trained on vertical-specific data will deliver significantly higher accuracy for domains like healthcare, legal, and financial services. Emotional intelligence capabilities will continue advancing, with AI systems better recognizing and appropriately responding to customer sentiment through tone and language analysis. Integration with emerging technologies like augmented reality will create new application possibilities, particularly for field service and technical support scenarios. The white label AI ecosystem will likely see consolidation among providers while simultaneously experiencing expansion in specialized technology niches. Platforms offering seamless customer service solutions that blend AI with human capabilities will likely see the strongest growth as businesses seek balanced automation approaches rather than complete replacement of human interactions.
Comparing Telephony Infrastructure Options for White Label AI
The underlying telephony infrastructure represents a critical decision point for white label AI voice implementations. Several approaches exist, each with distinct advantages for different use cases. Traditional Twilio integration offers reliability and global reach but at premium pricing that impacts overall solution costs. Twilio alternatives have emerged providing comparable capabilities at more competitive rates, particularly attractive for high-volume implementations. Direct SIP trunking connections offer the lowest per-minute costs but require more technical configuration and management. WebRTC implementations provide browser-based calling capabilities without telephony infrastructure but limit reach to internet-connected devices. Some platforms offer hybrid approaches that select optimal connection methods based on call circumstances. Businesses implementing white label voice AI must carefully evaluate these options based on call volume projections, international requirements, existing telephony investments, and technical capabilities. The decision significantly impacts both implementation complexity and long-term operational costs, with potential differences of 30-60% in per-minute expenses depending on the selected approach.
Performance Metrics and Analytics for White Label AI Implementations
Measuring the effectiveness of white label AI deployments requires comprehensive analytics frameworks that track both technical performance and business outcomes. Sophisticated implementations monitor conversation completion rates (successful handling without human escalation), sentiment analysis scores (detecting customer frustration or satisfaction), and resolution efficiency (time to complete tasks). Business impact metrics typically include conversion rates for AI sales calls, cost-per-acquisition changes, average handle time reductions, and customer satisfaction scores. Advanced analytics dashboards provide real-time visibility into these metrics, allowing continuous optimization of AI behavior based on performance data. Some platforms now incorporate A/B testing capabilities for conversation flows, enabling data-driven refinement of scripts and responses. Call recording and transcript analysis tools help identify common customer questions or objections that might require script adjustments. These analytics capabilities represent a significant advantage over traditional call centers, where performance visibility often relies on manual sampling and quality assurance processes. The most effective white label AI implementations establish clear baseline metrics before deployment and track improvements across 30, 60, and 90-day intervals to quantify ROI precisely.
How to Select the Right White Label AI Partner
Choosing the optimal white label AI provider requires evaluating several critical factors beyond basic functionality. Voice quality represents a foundational consideration—natural-sounding synthesis with appropriate intonation significantly impacts customer perception. Customization depth determines how effectively the solution can reflect brand values and specialized processes. Integration capabilities with existing business systems like CRMs, scheduling platforms, and e-commerce platforms affect implementation complexity. Scalability considerations include both handling peak call volumes and supporting business growth over time. Pricing structures vary widely, with some providers charging per minute, others per successful conversation, and still others through flat subscription models. Support quality proves particularly important for white label implementations, as technical issues directly impact brand reputation. Companies should request detailed demonstrations with custom script samples and conduct limited trials before full deployment. The market offers numerous specialized options, from AI bot white label solutions to comprehensive AI phone services, each optimized for different business requirements. The selection process should involve stakeholders from operations, marketing, and IT to ensure all requirements are adequately addressed.
Implementation Roadmap: From Selection to Deployment
Successfully implementing white label AI solutions follows a structured path from initial selection through full-scale deployment. The process typically begins with needs assessment and use case definition, identifying specific processes ripe for AI enhancement. Provider evaluation follows, comparing technical capabilities, integration options, and pricing models across competitive offerings. Pilot program design creates limited-scope implementations to validate performance before broader deployment. The customization phase involves script development, voice selection, and workflow definition—often the most time-intensive step in the process. Integration setup connects the AI platform with existing business systems, establishing data flows and handoff protocols. Testing and validation confirms functionality across various scenarios and edge cases. Agent training prepares human staff to work alongside AI systems, understanding escalation processes and handoff protocols. Phased rollout gradually expands implementation scope while monitoring performance. Post-deployment optimization uses analytics data to refine conversation flows and response patterns. This methodical approach mitigates risk while accelerating time-to-value. Companies implementing AI appointment setting or AI cold calling typically complete this process in 4-8 weeks, depending on customization complexity and integration requirements.
The Competitive Advantage: How White Label AI Transforms Business Models
White label AI creates distinctive competitive advantages that transform fundamental business operations and economics. The always-available nature of AI systems eliminates the concept of business hours, allowing 24/7 customer engagement without staffing costs or quality inconsistencies. Perfect scalability handles seasonal peaks or sudden demand surges without hiring challenges or quality degradation. The capability for perfect script adherence ensures regulatory compliance and consistent messaging across all customer interactions. The elimination of human limitations like fatigue, emotional responses, or knowledge gaps creates remarkably consistent customer experiences. Perhaps most significantly, the economic model fundamentally changes—businesses can grow customer interactions without proportional staffing increases, breaking the traditional linear relationship between revenue and support costs. Companies utilizing platforms like Callin.io for applications ranging from virtual call power to AI appointment booking have demonstrated the ability to scale operations rapidly while maintaining or improving customer experience metrics. This combination of operational flexibility, cost efficiency, and customer experience enhancement represents a structural advantage over competitors relying solely on traditional staffing models.
Unleash Your Business Potential with AI-Powered Communication
The white label AI revolution represents far more than a technological shift—it fundamentally changes what’s possible for businesses of all sizes. By removing the barriers of technical complexity and development costs, these solutions democratize access to sophisticated AI capabilities that were previously available only to enterprise organizations with substantial R&D budgets. Companies ranging from small professional practices to mid-sized regional businesses can now deliver communication experiences on par with global leaders. If you’re ready to transform your customer interactions with intelligent automation while maintaining your unique brand identity, Callin.io offers a comprehensive solution for implementing AI phone agents that handle appointments, answer questions, and even close sales through natural conversations. With the free account, you can explore the intuitive interface, configure your AI agent, and test calls immediately. For businesses ready to scale, premium plans starting at just $30 USD monthly provide advanced features like Google Calendar integration and CRM connectivity. Discover how easily you can implement this technology by visiting Callin.io today and joining the thousands of businesses already leveraging white label AI to create exceptional customer experiences.
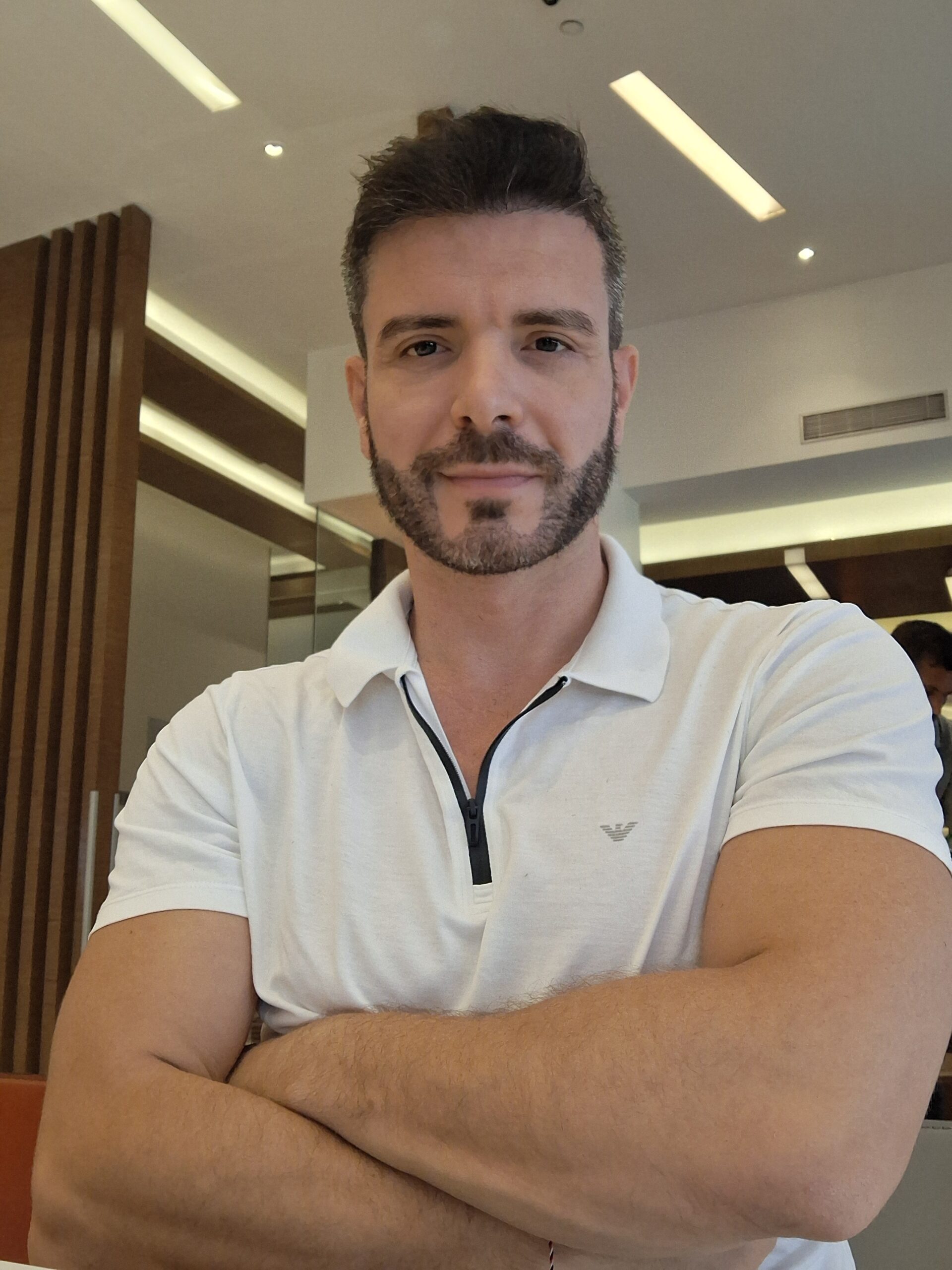
Helping businesses grow faster with AI. 🚀 At Callin.io, we make it easy for companies close more deals, engage customers more effectively, and scale their growth with smart AI voice assistants. Ready to transform your business with AI? 📅 Let’s talk!
Vincenzo Piccolo
Chief Executive Officer and Co Founder